In the age of Big Data, analysts are presented with billions of readily available data points and time series which can be used to identify trends, optimise processes, and efficiently manage risk. At Prescient Investment Management (PIM), we are no strangers to Big Data, processing over 120 million indicators per day. Our systematic, data-driven approach allows us to analyse these vast amounts of data, construct models, and derive insights to drive investment decisions.
Pictured : Sajjaad Ahmed, Portfolio Manager and Siphokuhle Zwane, Quantitative Analyst at Prescient Investment Management
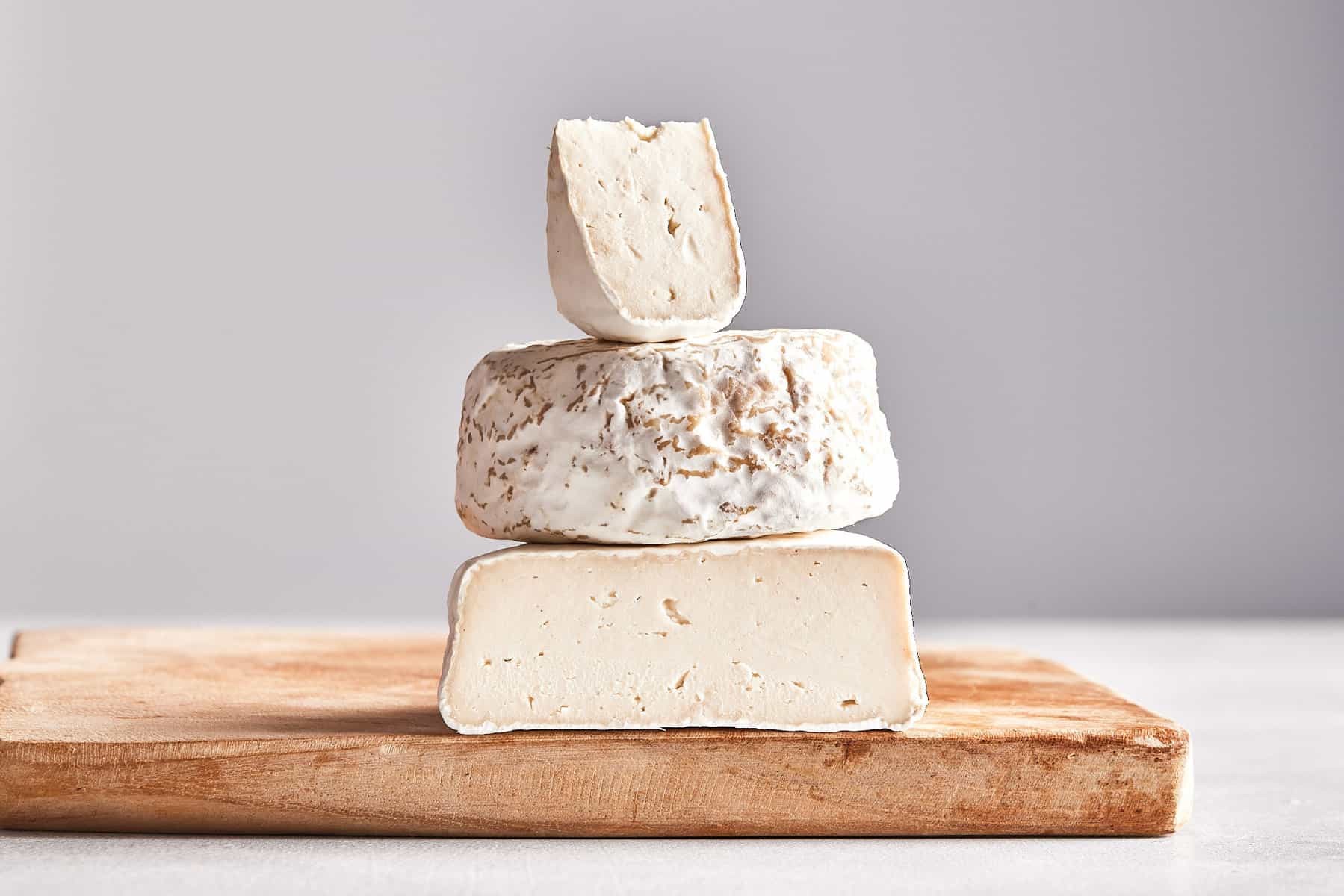
While it might sound like a good idea to throw the kitchen sink at trying to forecast the one-year ahead return on an asset class, one should take care to avoid spurious correlations. Simply put, if one time series appears to drive or influence another and they share a high correlation, it merits a deeper investigation to determine if the relationship will continue for the foreseeable future and if there is a plausible explanation for the correlation.
We illustrate this with two examples.
Figures 1 and 2 below shows the total per capita consumption of American cheese varieties in the United States, compared to South Africa’s Government Debt-to-Gross Domestic Product (GDP) ratio.
Figures 3 and 4 are a further example, illustrating the global number of Veganuary (a January challenge promoting eating vegan for a month) signups compared to the FTSE/JSE Top 40 Index (South Africa’s largest listed companies).
At first glance, these charts reveal very promising trends, with both series closely tracking each other over time. However, it is crucial to statistically measure the relationship between these series when building models, rather than relying on a quick—and often misleading—visual inspection. By doing so, we ensure that the analysis is based on robust evidence, providing more reliable insights and predictions.
In both examples, we consider two basic measures.
1. The “Pearson correlation” is often one of the first ways in which an analyst would measure a linear correlation. We calculate a correlation coefficient of 0.92 in example 1 and 0.95 in example 2. Note - a value of 1 indicates a perfectly positive correlation and thus the output of the above show that something is indeed happening here.
2. We can also plot a linear trend line through the data and find that:
a. The change in cheese consumption “explains” 85% of the variance, or change, in the debt-to-GDP ratio.
b. The change in Veganuary signups “explains” 90% of the variance, or change, in the FTSE/JSE Top 40 index.
As an importer of dairy products, one could posit that increased cheese consumption in the US would cause a surge in global dairy market prices, and that the higher import costs faced by South Africa would strain the national budget, leading to increased borrowing.
One could similarly argue that a surge in global Veganuary signups has driven technological advancements in farming methods and logistics due to the increased demand for organic food. These advancements, in turn, enhance global agricultural efficiency and transportation, leading to increased revenues and cost savings for companies. Consequently, it is reasonable to infer that such financial gains could lead to rising stock prices, thereby positively impacting indices such as the FTSE/JSE Top 40 Index.
Taking it back to the point of thorough analysis, delving deeper into these relationships is crucial because correlations can be deceptive. They might arise due to chance, external factors, or sheer coincidence. For investors, understanding whether a correlation is genuine or spurious is vital. A spurious correlation can lead to misguided decisions and potential financial loss.
Cause-and-effect relationships
In addition to the above, it is essential to recognise that correlation - two variables moving in tandem - does not imply causation (one variable causes the other to move). Statistical techniques primarily capture underlying patterns in data, rather than establishing cause-and-effect relationships.
Investors must also try to gauge causation. While two variables might move together, it is essential to determine if this co-movement is due to a real underlying relationship or merely coincidental. This involves rigorous analysis to assess whether one variable can reliably forecast or drive changes in another. Establishing causation helps in creating models that are not only accurate but also reliable over time.
Therefore, when evaluating correlations, investors should look for a plausible explanation and consider factors like economic theory, market mechanics, and historical context. This approach ensures that their forecasts are based on robust and meaningful relationships, rather than on mere statistical artifacts.
Additionally, understanding causation allows for better risk management and more informed decision-making, ultimately leading to more consistent investment outcomes.
What then?
A robust process for considering the inclusion of certain factors and/or variables is key, especially in PIM’s role as custodian of our clients’ hard-earned savings, where risk management is paramount. We take multiple steps to ensure that our systematic process is robust, allowing us to reduce human biases with unparalleled efficiency.
The first of these steps is to ensure we have the necessary expertise in our field to understand the context of the process, as well as to assess if the relationships make sense within the specified context. Any series being considered first undergoes a data cleaning process, warranting that the data is accurate, complete, and ready for model-building. At this stage, regularisation allows for the penalisation of overly complex models that capture noise rather than the underlying trend, favouring parsimonious models. We split the data into training and testing sets to validate the findings. The final model undergoes rigorous discussion and evaluation by the team to ensure that human biases and spurious correlations are effectively reduced.
Conclusion
It is our view that there is a key difference between testing anything-and-everything or implementing a thoughtful and systematic approach. In the age of big data, harnessing such power is essential for delivering long-term outperformance while placing risk at the heart of this process. This is what we do at PIM.
While we believe in the power of Big Data and the opportunities presented by it, sound data science and analytical methods allowing for the careful consideration of each metric are key to delivering consistent, long-term outperformance.
It is through this that asset managers will be able to efficiently navigate evolving markets, and uncertainty, and ultimately provide value to their clients.
Disclaimer:
Prescient Investment Management (Pty) Ltd is an authorised Financial Services Provider (FSP 612).
Please note there are risks involved in buying or selling a financial product, and past performance of a financial product is not necessarily a guide to future performance. The value of financial products can increase as well as decrease over time, depending on the value of the underlying securities and market conditions. There is no guarantee in respect of capital or returns in a portfolio. No action should be taken on the basis of this information without first seeking independent professional advice. Graphs are provided for illustrative purposes only.
*FAIS representative acting under supervision.
This document is for information purposes only and does not constitute or form part of any offer to issue or sell or any solicitation of any offer to subscribe for or purchase any particular investments. Opinions expressed in this document may be changed without notice at any time after publication. We therefore disclaim any liability for any loss, liability, damage (whether direct or consequential) or expense of any nature whatsoever which may be suffered as a result of or which may be attributable directly or indirectly to the use of or reliance upon the information. For more information visit www.prescient.co.za